일 | 월 | 화 | 수 | 목 | 금 | 토 |
---|---|---|---|---|---|---|
1 | 2 | 3 | 4 | 5 | ||
6 | 7 | 8 | 9 | 10 | 11 | 12 |
13 | 14 | 15 | 16 | 17 | 18 | 19 |
20 | 21 | 22 | 23 | 24 | 25 | 26 |
27 | 28 | 29 | 30 |
Tags
- Roc curve
- SMTP
- 트러블슈팅
- nvidia
- pandas
- nvidia-smi
- cuda
- semi-project
- 파일입출력
- 머신러닝
- selenium
- beautifulsoup
- 크롤링
- PYTHON
- Logistic linear
- 이것이 코딩 테스트다
- category_encoders
- AI
- Trouble shooting
- aof
- 잡담
- IOPub
- EarlyStopping
- 인공지능
- auc
- 그리디
- Django
- nvcc
- json
- ML
Archives
- Today
- Total
개발 블로그
K-Means algorithm 본문
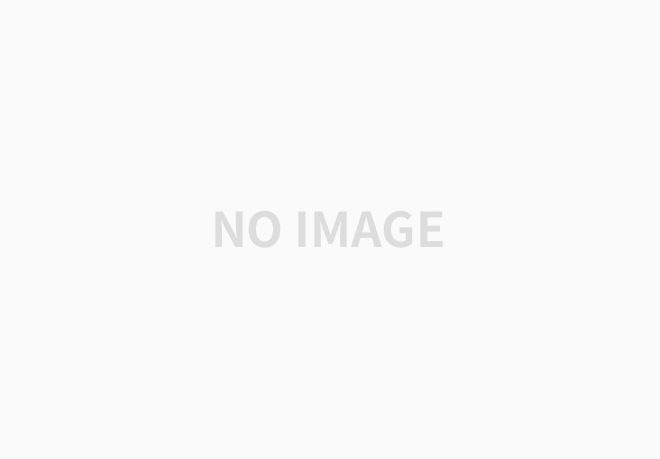
더보기
목차
01 K-Means algorithm
k-평균 알고리즘(K-means clustering algorithm)은 주어진 데이터를 k개의 클러스터로 묶는 알고리즘으로, 각 클러스터와 거리 차이의 분산을 최소화하는 방식으로 동작한다. 이 알고리즘은 자율 학습의 일종으로, 레이블이 달려 있지 않은 입력 데이터에 레이블을 달아주는 역할을 수행한다.
(https://ko.wikipedia.org/wiki/K-평균_알고리즘)
Algorithm
- K개의 임의의 중심값을 고른다. (보통 데이터 샘플 중의 하나를 선택)
- 각 데이터마다 중심값까지의 거리를 계산하여 가까운 중심값의 클러스터에 할당한다.
- 각 클러스터에 속한 데이터들의 평균값으로 각 중심값을 이동시킨다.
- 데이터에 대한 클러스터 할당이 변하지 않을 때까지 2와 3을 반복한다.
- 단점
- 연산시간이 오래 걸린다.
- 이상값에 민감하다.
01_01 실습 (K-Means for Iris data)
sclearn에서 제공하는 iris dataset을 이용해서 K-Means를 구해봅니다.
import numpy as np
import matplotlib.pyplot as plt
from mpl_toolkits.mplot3d import Axes3D
from sklearn import cluster
from sklearn import datasets
from sklearn import metrics
iris = datasets.load_iris()
X = iris.data
y = iris.target
print(X)
print()
print(y)
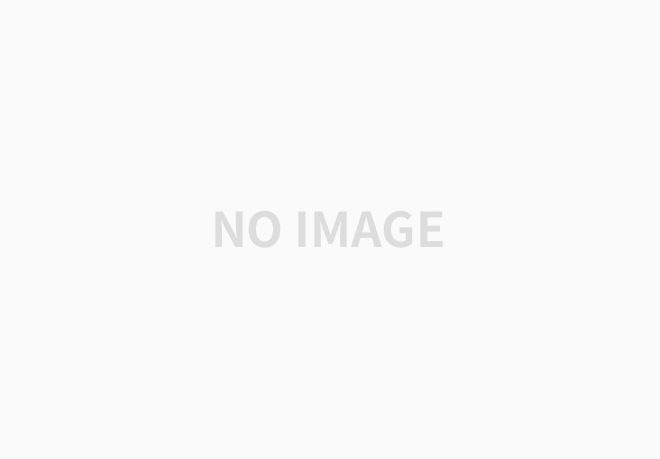
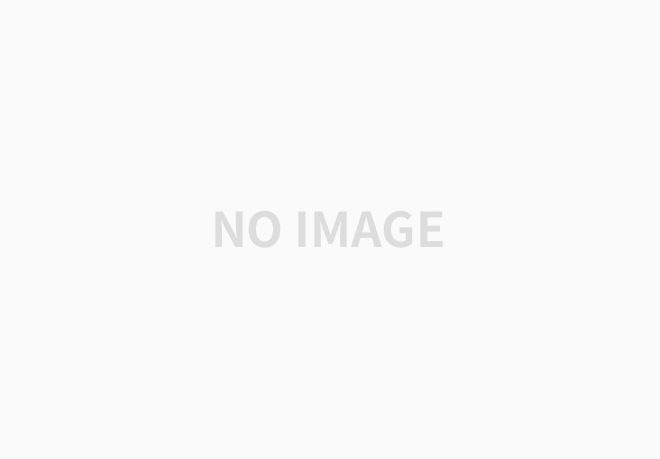
3가지 경우의 K-Means를 구합니다.
첫번째는 k값이 8,
두번째는 k값이 3,
세번째는 k값이 3이면서 init='random' parameter를 추가 하였습니다. init의 default값은 k-means++입니다.
estimators = [('k=8', cluster.KMeans(n_clusters=8)),
('k=3', cluster.KMeans(n_clusters=3)),
('k-3', cluster.KMeans(n_clusters=3, n_init=1, init='random'))]
print(estimators[0])
print(estimators[1])
print(estimators[2])
시각화
fignum = 1
titles = ['8 clusters', '3 clusters', '3 clusters, bad initialization']
for name, est in estimators: # estimators : ('k=8', cluster.KMeans(n_clusters=8))
fig = plt.figure(fignum, figsize=(7, 7)) # fignum 도화지를 여러장 만든다
ax = Axes3D(fig, elev=48, azim=134) # Set the elevation and azimuth of the axes. (축의 고도와 방위각)
est.fit(X)
labels = est.labels_
# X = iris.data
ax.scatter(X[:, 3], X[:, 0], X[:, 2], c=labels.astype(np.float), edgecolor='w', s=100)
ax.w_xaxis.set_ticklabels([])
ax.w_yaxis.set_ticklabels([])
ax.w_zaxis.set_ticklabels([])
ax.set_xlabel('Petal width')
ax.set_ylabel('Sepal length')
ax.set_zlabel('Petal length')
ax.set_title(titles[fignum - 1])
ax.dist = 12 # 값이 커지면 전체 plot 이 작아짐
fignum = fignum + 1
plt.show()
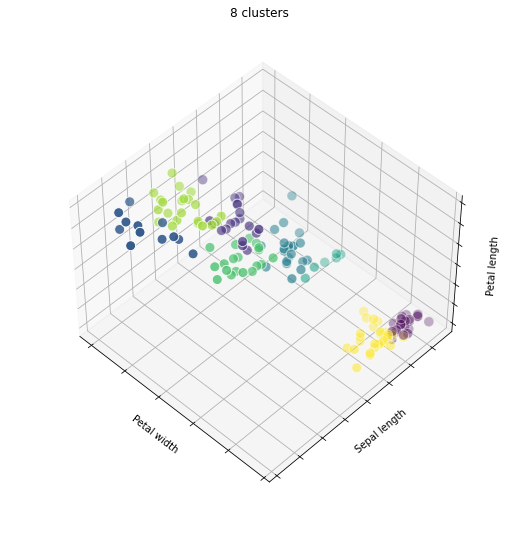
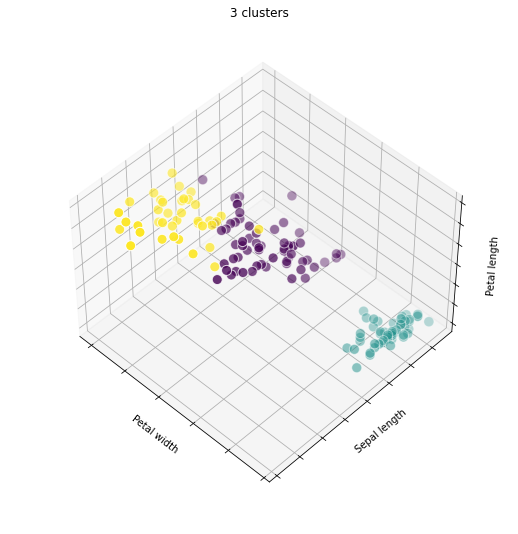

# Plot the ground truth (gt : 원본, 정답을 의미)
fig = plt.figure(figsize=(7, 7))
ax = Axes3D(fig, elev=48, azim=134)
for name, label in [('Setosa', 0), ('Versicolour', 1), ('Virginica', 2)]:
ax.text3D(X[y == label, 3].mean(), X[y == label, 0].mean(), X[y == label, 2].mean()+2,
name, horizontalalignment='center')
ax.scatter(X[:, 3], X[:, 0], X[:, 2], c=y, edgecolor='w', s=100)
ax.w_xaxis.set_ticklabels([])
ax.w_yaxis.set_ticklabels([])
ax.w_zaxis.set_ticklabels([])
ax.set_xlabel('Petal width')
ax.set_ylabel('Sepal length')
ax.set_zlabel('Petal length')
ax.set_title('Ground Truth')
ax.dist = 12
plt.show()
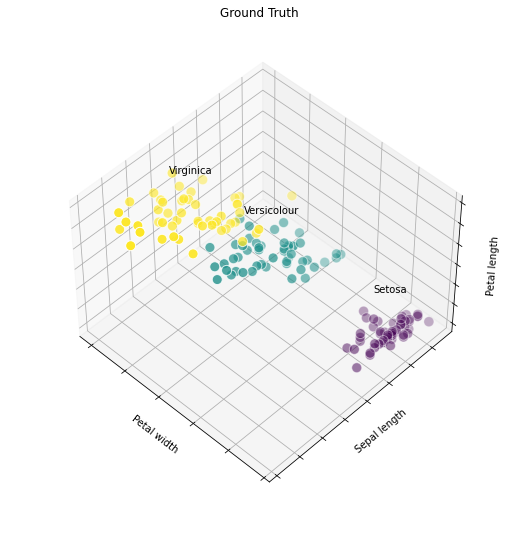
02 최적 클러스터 개수 찾기
02_01 elbow 기법
- 클러스터의 수를 순차적으로 늘려가면서 결과를 모니터링 한다. 만약 하나의 클러스터를 추가했을 때 이전보다 훨씬 더 나은 결과를 나타내지 않는다면, 이전의 클러스터의 수를 구하고자 하는 클러스터의 수로 설정한다.
- 결과물인 그래프 모양을 보면 팔꿈치에 해당하는 바로 그 부분이 최적의 클러스터 개수가 됨.
def elbow(X):
total_distance = []
for i in range(1, 11):
model = cluster.KMeans(n_clusters=i, random_state=0)
model.fit(X)
# inertia : Sum of squared distances of samples to their closest cluster center.
total_distance.append(model.inertia_)
plt.plot(range(1, 11), total_distance, marker='o')
plt.xlabel('# of clusters')
plt.ylabel('Total distance (SSE)')
plt.show()
elbow(X) # Iris case : 2
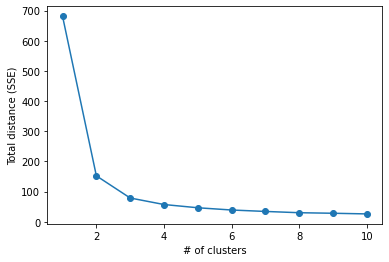
02_02 실루엣 기법
- 클러스터링의 품질을 정량적으로 계산해주는 방법 (K-means 뿐만 아니라 모든 클러스터링 기법에 적용 가능)
- i번째 데이터 x(i)에 대한 실루엣 계수(silhouette coefficient) s(i) 값은 아래의 식으로 정의
- a(i)는 클러스터 내 데이터 응집도(cohesion)를 나타내는 값 == 데이터 x(i)와 동일한 클러스터 내의 나머지 데이터들과의 평균 거리
- b(i)는 클러스터 간 분리도(separation)를 나타내는 값 == 데이터 x(i)와 가장 가까운 클러스터 내의 모든 데이터들과의 평균 거리
- 만약 클러스터 개수가 최적화 되어 있다면 b(i)의 값은 크고, a(i)의 값은 작아짐 -> s(i)의 값은 1에 가까운 숫자가 됨
- 반대로 클러스터내 데이터 응집도와 클러스터간 분리도의 값이 같으면 실루엣 계수 s(i)는 0 (데이터들을 클러스터로 분리하는 것이 무의미)
- 요약) 클러스터의 개수가 최적화되어 있으면 실루엣 계수의 값은 1에 가까운 값이 됨
import numpy as np
from sklearn.metrics import silhouette_samples
from matplotlib import cm
def plotSilhouette(X, y_fitted):
cluster_labels = np.unique(y_fitted)
n_clusters = cluster_labels.shape[0] # ex) (3,) -> 3
silhouette_vals = silhouette_samples(X, y_fitted, metric='euclidean') # y_fitted 클러스터 라벨을 기준으로 한 X 데이터 각각이 가지는 실루엣 계수를 계산
y_ax_lower, y_ax_upper = 0, 0
yticks = []
for index, label in enumerate(cluster_labels):
cluster_silhouette_vals = silhouette_vals[y_fitted == label] # 각 라벨(center=3이면 0,1,2)에 해당하는 예측 데이터들의 실루엣 계수
cluster_silhouette_vals.sort()
# 라벨 순서대로 클러스터로 할당된 데이터 수만큼 y_ax_upper 에 더하여 y축 방향으로 쌓음
y_ax_upper += len(cluster_silhouette_vals)
plt.barh(range(y_ax_lower, y_ax_upper), cluster_silhouette_vals, height=1.0) # barh(y, data), edge_color=None
yticks.append((y_ax_lower + y_ax_upper) / 2) # 그래프에서 y축 위에 클러스터 번호 라벨링 적용
# 라벨 순서대로 클러스터로 할당된 데이터 수만큼 y_ax_lower 에 더하여 y축 방향으로 쌓음
y_ax_lower += len(cluster_silhouette_vals)
silhouette_avg = np.mean(silhouette_vals) # 전체 데이터에 대한 실루엣 계수의 평균
plt.axvline(silhouette_avg, color='red', linestyle='--') # 전체 데이터에 대한 실루엣 계수의 평균을 수직선으로 표시
print('The average silhouette value is', round(silhouette_avg, 2), '(near 0.7 or 0.7+ : desirable)')
plt.yticks(yticks, cluster_labels+1)
plt.ylabel('Cluster')
plt.xlabel('Silhouette value')
plt.show()
model = cluster.KMeans(n_clusters=2) # Change the number of clusters
y_fitted = model.fit_predict(X)
plotSilhouette(X, y_fitted)
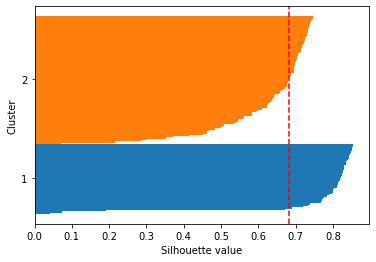
'AI' 카테고리의 다른 글
Deep Learning이란? (0) | 2022.04.27 |
---|---|
Logistic Regression & Cross-entropy function & ROC Curve, AUC (0) | 2022.04.24 |
Linear Regression(선형 회귀) & Gradient Descent Algorithm(경사하강법) (0) | 2022.04.15 |
PCA ( Principal Component Analysis) (0) | 2022.04.13 |
인공지능과 머신러닝의 개념과 분류 (0) | 2022.04.13 |